Deepseek R1 has gained attention for its impressive reasoning capabilities, but it is essential to view it as an evolution rather than a revolution. While some discussions frame R1 as a paradigm shift, the reality is that it represents a refinement of existing AI training methodologies rather than a fundamental change in how AI models operate.
The key innovation in Deepseek R1 lies in its training approach. Unlike previous iterations of large language models, R1 leverages reinforcement learning to address known challenges in model training. This adjustment has significantly improved its reasoning capabilities, making it stand out among competitors. However, the underlying model architecture remains largely unchanged. The difference is in how Deepseek optimized the training process, demonstrating that strategic refinements can yield significant improvements. This has surprised the industry, not because such progress was unforeseen, but because Deepseek executed it efficiently and at a competitive cost.
This advancement highlights an important reality: AI development is an ongoing process, with continuous refinements leading to better models. Deepseek’s success illustrates how AI researchers can push the boundaries of machine learning without necessarily requiring massive computational scale-ups. Instead, better training techniques can enhance performance in meaningful ways.
"AI innovation isn’t about making machines think like us—it’s about expanding what’s possible, pushing boundaries, and redefining how we solve problems."
- Sumit Chaturvedi, Entrepreneur, Global Consulting
Another point of discussion surrounding R1 is its moderation policies and data usage. Like all AI models, Deepseek R1 operates within an ethical and regulatory framework that shapes what it can and cannot generate. Content restrictions are not unique to R1, as all major AI providers implement guidelines based on legal, ethical, and corporate considerations. Additionally, concerns about data retention and usage should be examined in the broader context of AI governance rather than being attributed solely to specific models or companies.
The broader implication of Deepseek R1’s success is that AI development remains a dynamic and rapidly evolving field. The launch of R1 reinforces that innovation is not slowing down and that there is still significant room for optimization in AI training methodologies. More importantly, it demonstrates that advancements in AI do not always require drastically larger models; instead, they can stem from smarter training techniques that improve efficiency and performance.
Rather than focusing on whether R1 represents a revolutionary shift, the more meaningful discussion is about
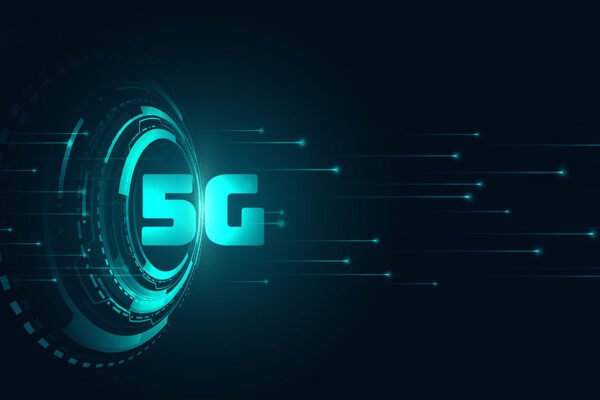
- How AI continues to progress
- What these developments mean for the future of the field
- How new iteration of AI technology, contributes to a more competitive and innovative landscape
- How does it impacts the benefiting researchers, developers, and users alike.
Deepseek R1 is a reminder that AI remains in its early stages, with much more room for growth. Instead of framing every new advancement as a dramatic disruption, it is more productive to recognize the incremental yet impactful progress being made. R1 is a step forward—one that highlights the potential for continued breakthroughs in AI research and development.